Introduction: A New Era of Data-Driven, AI-Powered Research
Biotech laboratories in 2025 are managing more data and complexity than ever before. Annual data generation in life sciences is exploding, projected to reach up to 40 exabytes per year by 2025. Organizations are racing to leverage this information for competitive advantage. At the same time, emerging technologies like artificial intelligence (AI) and lab automation are becoming central to R&D and operations, driving an 8%+ annual growth in the lab informatics market.
Lab executives face a dual challenge: enable cutting-edge, data-driven science (often assisted by AI) while maintaining rigorous compliance for any workflows under GxP (Good Practice) regulations. They need platforms that can flex for early discovery and also lock down for regulated processes, all without creating silos or losing control of critical data. In this context, many are re-evaluating legacy electronic lab notebooks (ELNs) and LIMS solutions that were not designed for the volume, velocity, and variety of today's lab data.
Executives and lab managers are asking: What kind of laboratory data platform can truly support the modern, AI-augmented lab that runs both exploratory research and compliant manufacturing without compromise? This post examines why Scispot has emerged as that platform of choice for forward-looking labs in 2025. We take an analytical look at the requirements of today's laboratories, the shortcomings of older lab informatics systems, and Scispot's key differentiators that uniquely position it to meet the needs of data-intensive, automated, and compliance-conscious environments.
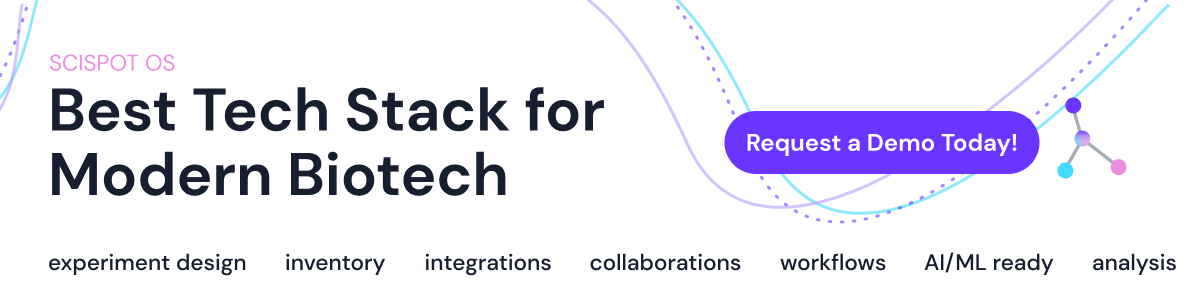
The Evolving Needs of Modern Labs
Modern R&D and production labs are increasingly data-driven and automated. Scientists and analysts expect to capture data from every instrument in real-time, integrate results across experiments, and apply AI/ML models to glean insights. According to industry research, adoption of AI and lab automation has become a key driver of lab informatics investments.
Labs now require:
Seamless Digital Data Capture: Every assay, instrument reading, and protocol detail should flow into a central repository automatically, reducing manual transcription. This demands broad integration capabilities for instruments and software.
Advanced Analytics and AI Integration: Teams want to analyze data in situ using tools like Jupyter notebooks, and even query datasets with AI assistants. The platform must be AI-ready, enabling everything from simple data querying to running machine learning workflows on laboratory data.
Flexible Yet Structured Workflows: In early research, scientists need flexibility to innovate. Quick database adjustments, new experiment types, and rapid iteration are essential. In later-stage or clinical workflows, the same platform must enforce structured processes with audit trails and approvals (GxP compliance). The ideal system should seamlessly support both exploratory and regulated workflows.
Extensibility and Avoiding Lock-In: Life science innovation moves fast, so labs demand a system that can evolve through APIs, custom plugins, or connectors rather than a closed "black box." Crucially, they want full control of their data so they're not handcuffed to a vendor. Exporting or backing up data to internal data lakes or warehouses should be straightforward.
Scalability and Cost-Effectiveness: As a biotech scales from a small team to an enterprise, the informatics platform must scale without painful migrations or exorbitant cost. Labs seek transparent, predictable pricing that won't spike with each additional user or dataset, and a solution that can grow from a few users to hundreds without a complete overhaul.
User-Friendly Experience and Support: Lastly, adoption by scientists (not just IT staff) is critical. A modern UI and white-glove support for onboarding and optimization can make the difference between a system that sits idle and one that becomes mission-critical.
In short, the lab of 2025 needs a unified data platform that is flexible, AI-enabled, highly integrative, compliance-ready, and customer-centric. How do legacy lab informatics solutions stack up on these requirements?
Limitations of Legacy Lab Data Platforms
Many incumbent ELN/LIMS platforms in use today were built in a different era and often struggle to meet the above needs. These older, monolithic systems tend to be rigid and inflexible, forcing labs to conform their processes to the software rather than the other way around. It's common for scientists to have to significantly modify workflows or even write custom SQL code to fit a legacy LIMS, often requiring vendor professional services for configuration.
This not only slows down implementation, but any change in the lab's process (a new assay, a new data type) becomes a heavy lift. By contrast, modern approaches emphasize no-code or low-code configurability so that labs can adapt the software themselves in real-time. For example, Scispot uses a no-code configuration approach that allows labs to adjust workflows without programming, slashing typical implementation timelines to 6–12 weeks versus the many months legacy systems often require.
Another pain point with traditional platforms is integration and data siloing. Legacy LIMS/ELNs often have limited out-of-the-box integrations, maybe supporting a few major instruments or requiring custom development for each new connection. As a result, labs end up with fragmented systems, one for instrument data, one for inventory, another for analysis, that don't talk to each other.
Manual data transfer or tedious CSV imports/exports become routine, introducing errors and wasting time. Worse, some vendors make it intentionally difficult to export data in bulk, leading to "data lock-in" where migrating off the platform is painful. An executive of one biotech famously likened a leading LIMS vendor to a "Ticketmaster of biotech software," charging high fees and "nickel-and-diming" for help, while providing limited data portability. Such vendor lock-in poses strategic risks for labs that need agility in their data management.
Cost and scalability are additional concerns. Traditional enterprise LIMS licenses can run into the thousands of dollars per user per year, an untenable model for startups or mid-size companies trying to scale. And if a lab outgrows the system's capacity or needs new functionality, often the only path is a major system overhaul or replacement, since older systems weren't designed to expand modularly. This "rip and replace" approach is the last thing a growing biotech wants to deal with in the middle of scaling operations.
Legacy vendors with on-premises architectures also struggle to match the elastic scalability of cloud-native solutions, resulting in either slow performance or costly infrastructure upgrades as data volumes increase. Finally, while legacy LIMS vendors do offer compliance modules, they are often add-ons that come with complexity. Many older platforms have outdated user interfaces that hamper user adoption, and support may be limited to generic documentation or long ticket queues. In regulated settings, the lack of real-time support can be problematic when an issue arises during an audit or validation.
In summary, traditional lab informatics platforms tend to be rigid, slow to implement, integration-poor, expensive, and prone to vendor lock-in. They served well in the past for basic sample tracking or documentation, but they are mismatched to the dynamic, data-heavy workflows of 2025. Laboratories are increasingly seeking a new solution, one that combines the best of an ELN, LIMS, and data science platform in a flexible, scalable package. This is exactly the void that Scispot was designed to fill.
Scispot's Differentiators: A Platform Built for Modern Lab Needs
Scispot brands itself as a unified Lab Operating System (LabOS) rather than just an ELN or LIMS. In practice, it combines the functionalities of an ELN, LIMS, and scientific data management system (SDMS) on a cloud-based, API-first architecture. Below, we break down how Scispot's approach addresses the shortcomings of legacy systems and delivers on the requirements of modern data-driven labs.
.png)
API-First Architecture with a Data Lake Foundation
At the core of Scispot is an API-first design and a built-in scientific data lake. Every feature of the platform is accessible via APIs, which means labs can programmatically interact with their data and workflows. This gives organizations full technical control to integrate or extract data at will and to automate tasks via code. Researchers and developers can, for instance, write scripts to design custom databases, configure instruments, or trigger analyses, all through Scispot's API layer.
In contrast to closed systems, this open architecture ensures that your data is never trapped: if you need to push experimental data to an in-house database or pull results into a visualization tool, it's straightforward. In fact, developers can even maintain a replica of all Scispot data in the lab's own cloud storage (AWS S3, Google Cloud, Azure), retaining full ownership and redundancy. This level of data control virtually eliminates the risk of vendor lock-in, since the lab always has a current copy of its data and the means to work with it outside the platform if needed.
Crucially, Scispot's back-end is organized as a scientific data lakehouse (their "alt-SDMS"), not just a rigid relational database. Unlike legacy SDMS products that act as passive data vaults, Scispot's data lake approach ingests raw instrument files, structured records, and metadata in real-time and makes them immediately available for query and analysis. This means that whether you're capturing genomic sequences from a sequencer or images from a high-content microscope, the data lake can store it in native form and apply transformations on the fly.
The benefit is twofold: all lab data gets unified (no more scattered silos of spreadsheets and servers), and it's instantly "analytics-ready" for AI processing. As Scispot describes, their SDMS isn't just storage, it's an active data refinery that turns raw data into usable insights via built-in pipelines and AI analytics. For lab managers, this translates to less time wrangling data and more time extracting value from it.
Another advantage of an API-first, cloud-native architecture is scalability. Scispot can scale storage and compute resources elastically as the lab's data grows, without performance degradation. A startup can start small and, as data volumes surge from megabytes to terabytes, the platform scales behind the scenes, avoiding the nasty surprises of hitting a database size limit or slowing to a crawl.
This future-proof design ("built for growth") means a lab won't need to switch systems as it expands; one system can serve from R&D startup phase through clinical trial phases to commercial manufacturing. Indeed, Scispot's modular LabOS allows incremental expansion, providing "the rare combination of startup flexibility with enterprise-grade scalability," unlike traditional systems that often require a full replacement to handle scale.
Built-in AI and Workflow Automation Tools
A defining feature of Scispot's platform is its native AI and computation layer. Instead of treating analysis and automation as external activities, Scispot embeds these capabilities right into the LabOS. For example, it includes an AI-driven Lab Assistant (dubbed "Scibot") that leverages large language models to allow users to interact with their lab data in natural language.
In practical terms, a scientist can ask the system a question, e.g., "Which formulation had the highest stability in last quarter's experiments?" and the AI will fetch and analyze the relevant data from the ELN/LIMS records to provide an answer. This turns what used to be multi-click, multi-step database queries into a conversational experience. Labs have reported that routine processes are streamlined from many manual steps into a single AI chat interaction.
The AI assistant isn't just for Q&A; it can also automate repetitive tasks (like setting up experiment templates, initiating workflows, or even generating summary reports), effectively acting as a productivity multiplier for lab staff. Complementing the AI assistant, Scispot has an embedded computational environment for more technical users. Data scientists or computational biologists can launch Jupyter notebooks or RStudio sessions within Scispot, running Python or R analyses directly on lab datasets without switching context.
This is a major leap from older LIMS, where one would export data to a separate environment for analysis. Here, users can do everything from statistical analysis, custom visualizations, to machine learning model training on the platform. Because the data is already centralized in the Scispot data lake, notebooks can pull live data, perform analysis, and even push results or figures back into the ELN as records, creating a closed-loop between experimentation and analysis. Scispot's integration of JupyterHub for code-first users and no-code AI tools for others means it caters to the full spectrum of staff, from lab technicians to bioinformaticians.
The platform's AI capabilities also extend to automated analytics and dashboards. A recent update allows users to simply describe the chart or report they want, and the system's AI (Scibot) will generate interactive dashboards on the fly. For instance, a lab manager could ask for a dashboard of weekly instrument utilization or reagent consumption, and the AI will produce it without any coding or manual data wrangling. These kinds of AI-driven features are increasingly important as labs strive to be more data-driven in decision-making. Scispot essentially bakes in an AI analytics platform on top of the raw data, replacing the need for separate BI tools or manual spreadsheet analysis.
By having automation and AI inherently available, Scispot helps labs not only collect data but actually derive insights and efficiencies from that data. Whether it's an algorithm spotting an anomaly in an assay result or an AI assistant auto-completing an experiment write-up, these tools save scientists time and augment their capabilities. Legacy systems that lack such features force labs to bolt on third-party analytics or live without those insights. In contrast, Scispot positions AI as a first-class component of the lab workflow, aligning with where the industry is headed.
Seamless Integration with Instruments and Apps (No More Data Silos)
One of Scispot's most touted strengths is its extensive integration ecosystem. Modern labs might use dozens of instruments and software applications, from electronic pipettes and bioreactors to LIMS, ELNs, inventory systems, analysis software, and more. Scispot addresses this complexity with its proprietary integration engine (called GLUE), which offers plug-and-play connectivity to thousands of tools.
Specifically, Scispot can connect to over 200 different lab instruments and 7,000+ software applications via pre-built connectors and APIs. This breadth is far beyond the typical handful of integrations provided by legacy LIMS out-of-the-box. It means that common lab devices like HPLC machines, mass spectrometers, plate readers, flow cytometers, etc., can stream data directly into Scispot in real-time. Likewise, popular software and databases (from CRM systems to data analysis platforms) can be linked so that information flows without manual intervention.
The impact of this is significant: data capture becomes largely automatic. For example, when a scientist runs a sample on a chromatography system, the result file can be ingested by Scispot immediately, tagged to the correct sample record in the LIMS, and made available for review or analysis. There's no waiting to upload files or transcribe results, and the integration ensures consistency (reducing human error). This real-time, integrated data pipeline provides end-to-end visibility of experiments and operations. Lab managers can monitor experiment status or instrument outputs as they happen, enabling faster decision-making. Scispot's integrative approach essentially eliminates the data islands that many labs struggle with, instruments no longer sit in isolation but rather feed a unified data hub.
Another advantage of Scispot's integration-first philosophy is future-proofing and avoiding vendor lock-in. Because it's built to connect with external systems (even other LIMS or ELNs), labs can use Scispot as a central orchestrator without having to abandon every legacy tool immediately. For instance, if a lab still likes a particular analysis software, they can integrate it rather than being forced to use only Scispot's analytics. Similarly, Scispot can push data to external databases or data lakes in the company's IT environment, aligning with an enterprise's broader data strategy.
This interoperability ensures that adopting Scispot doesn't mean jettisoning all existing IT investments, instead, it can enhance them by filling the integration gaps. In fact, Scispot's GLUE engine has been used to interface with other major lab platforms (LabWare, Benchling, etc.) when companies operate hybrid environments, demonstrating an openness rarely seen in the industry. The message to customers is clear: your data will not be chained inside Scispot, you're free to integrate and migrate as you see fit.
From a cost and efficiency standpoint, having 200+ instrument integrations ready out-of-the-box also saves labs potentially hundreds of thousands of dollars in custom development that would be needed with less extensible systems. And it speeds up implementation, labs don't have to wait months for a vendor to build a connector to their specific instrument model; chances are, Scispot already has it, or the API is available to build it quickly. This fits the modern lab operations ethos of automation: scientists should be doing science, not moving files between systems. Scispot's integration prowess removes a huge chunk of manual data wrangling from daily work, which is a key reason many fast-growing biotechs are gravitating to it.
Unified Platform for Both Research and GxP Workflows
Historically, companies have often used one set of tools for R&D and a different system for clinical or manufacturing work under GxP regulations. Scispot challenges that paradigm by providing a single platform that can seamlessly support both non-GxP and GxP workflows within the same environment. This is a significant differentiator for organizations aiming to streamline technology and avoid duplicate systems.
In exploratory research mode, Scispot acts as a flexible ELN/LIMS where scientists can quickly spin up new experiments, collaborate in real-time, and capture data without rigid formality. Its interface for experiment design and data capture is user-friendly, and the underlying data models can be configured on-the-fly to fit novel research workflows. This flexibility is key for early R&D teams that might be doing something new every week. Scispot allows them to mold the digital system to their science, rather than forcing the science into preset templates. Features like version-controlled notebooks, team comments, and internal knowledge sharing tools help maintain scientific context and foster collaboration in this phase.
When it's time to transition discoveries into regulated development and production, Scispot hardens into a full compliance platform. All the necessary GxP controls are built-in: 21 CFR Part 11-compliant electronic signatures and audit trails, strict role-based access control (RBAC), enforced versioning of records, and validation support. For example, every change to a protocol or dataset can be tracked with a timestamped audit log, and certain actions can require multi-step approval with e-signatures, meeting FDA and ISO requirements for data integrity and traceability.
Scispot is also compliant with standards like SOC 2, HIPAA, GDPR, and GLP/GMP requirements, indicating that it has the security and process controls needed for handling sensitive patient data or quality records. Essentially, the platform can operate in a validated state suitable for use in clinical trials or manufacturing QA/QC, which is often a gate for using any software in those contexts.
What makes Scispot unique is that it doesn't split these modes into separate products, the same platform can be used by an R&D scientist exploring a new hypothesis and by a QA associate doing a batch release review. This unification of R&D and GxP means data flows uninterrupted across the lifecycle. A method developed in research can be directly carried over to a validated protocol with appropriate permissions, retaining all the historical context.
There's no need to migrate data from an ELN to a separate LIMS when moving to preclinical or clinical phase, avoiding a common source of errors and miscommunication when using disparate systems. As the Scispot team puts it, it enables a "seamless transition" between discovery and development phases, improving efficiency and reducing the potential for error that arises from system hops.
For executives, this convergence can also yield cost savings and simpler IT management: one platform to qualify, one vendor to deal with, one training curriculum for staff. It's also future-proof from a regulatory standpoint, knowing that as the company's work becomes more regulated, the software will already be audit-ready. Labs can thus start using Scispot early (for its agility) and trust that it will support them later (for compliance), rather than having to abandon it for a big enterprise LIMS when they grow. This dual capability is a major competitive edge over older vendors that offer either flexibility or compliance, but not both in equal measure.

White-Glove Onboarding and Customer Support
Technology aside, Scispot differentiates itself with a very customer-centric service model, often described as "white-glove support." From the moment a lab signs on, Scispot provides personalized onboarding with hands-on assistance in setting up the system to the lab's specific needs. Each customer is assigned a dedicated account manager and even given a private Slack or Microsoft Teams channel for direct communication with Scispot's science and engineering team. This level of access is uncommon in the software industry, and it means that when a lab encounters a challenge or needs a new integration, they can get near-instant help from the people who build the product.
One user, a healthcare data analyst at a lab, noted that Scispot's team would assist with configuring custom workflows and answer questions "with near-instant responses on Slack," and found integrating instruments via Scispot's GLUE toolkit to be seamless with this support.
The benefit of such close support is faster time to value and higher user adoption. Lab staff can have experts guiding them through configuring templates, setting up databases, or importing legacy data. If something isn't working optimally, Scispot's team can often tweak or suggest a solution in real-time. This is in stark contrast to some traditional vendors who might take days to respond to support tickets, or charge professional service fees for customizations.
By embedding their support alongside the customer's team, Scispot ensures that the platform is truly tailored to each lab's processes, and that users are fully trained and comfortable. Ongoing consulting is also part of the package, as the lab's needs evolve, Scispot's team assists in optimizing workflows and implementing new features. Essentially, they partner in the lab's success rather than just delivering software and walking away.
For busy lab managers, this white-glove treatment reduces the risk of a failed implementation (a notorious problem with enterprise software). It also means internal IT resources can be minimal, you don't need a full-time LIMS administrator on staff when the vendor is actively helping configure and maintain the system. Many scaling biotechs find this attractive because they might lack extensive IT teams. Scispot's approach here appears to lower the barriers to entry that often come with adopting a comprehensive data platform.
Furthermore, Scispot's support extends beyond just technical issues, they actively help with compliance readiness (e.g., providing validation document support, advising on best practices to meet GxP requirements) and with data strategies (like how to structure your databases for optimal analysis). This consultative support is analogous to having a high-level expert for your lab digitization on call, which resonates well with executive-level buyers. The result is that labs can hit the ground running and see ROI faster, and they have a safety net if any hiccups occur.
Transparent Pricing and Full Data Ownership
Another area where Scispot breaks from legacy providers is in its business model transparency. Traditionally, lab software pricing could be opaque and filled with surprise add-on costs, e.g., extra fees for each integration module, API access, or even for retrieving your own data. Scispot has aimed for a more modern SaaS-style pricing that is usage-based and predictable.
They offer transparent pricing with volume-based discounts as the lab's operations grow. This means a company can plan its budget knowing that as they add more users or more data, the cost per unit may actually decrease at higher volumes, and there won't be hidden charges popping up. Such clarity is especially important for startups and scale-ups operating under tight budgets; no CFO wants to discover that adding a new team to the LIMS will blow the budget. By simplifying pricing, Scispot positions itself as a scalable partner, labs can start small and scale usage broadly without punitive costs, aligning with the goal of not letting software costs hinder scientific growth.
Perhaps even more critical is Scispot's stance on data ownership. In Scispot, the customer unequivocally owns their data, the platform is just the custodian and facilitator. We touched on the technical enablers of this (API access, ability to backup data to your own storage). From a policy perspective, Scispot does not lock customers in by withholding data or using proprietary formats that can't be exported.
All data in the system can be exported in standard formats via the API or built-in tools. In fact, as noted earlier, power users can set up continuous backups of all their records to their private cloud storage. This approach contrasts with legacy vendors where data export might be limited or require special requests (some labs have found it non-trivial to get their complete dataset out of older LIMS). For regulated labs, data ownership and traceability are not just preferences but often regulatory expectations, knowing you have full custody of your data and can retrieve it any time is important for compliance and peace of mind.
Moreover, Scispot's openness means labs avoid vendor dependency in the long run. They can integrate Scispot with a larger data architecture (like a company-wide data lake or ERP system) as a component, rather than creating another isolated data silo. Should the lab ever decide to change systems, their data can move with them. This philosophy is somewhat disruptive in the LIMS market, where historically switching costs were high.
But it aligns with the modern enterprise trend toward data democratization, the idea that data should flow to wherever it's needed and not be artificially siloed by applications. By not trapping data or charging ransom for it, Scispot builds trust with its customers. An executive can feel comfortable that adopting Scispot is not a one-way door; it's chosen on merit, and the data remains in the company's domain.
From the perspective of an analytical comparison, this customer-friendly model (transparent pricing + data ownership) addresses two major concerns that often come up in procurement discussions. It removes financial uncertainty and it removes fear of lock-in, making the decision to choose Scispot much easier to justify. In essence, Scispot is aligning its success with the customer's success, if the platform delivers value, the customer will keep using it (and paying the subscription), but they are free to leave if not, with their data intact. That dynamic incentivizes Scispot to continually provide value, service, and innovation to retain clients, which ultimately benefits the end users.
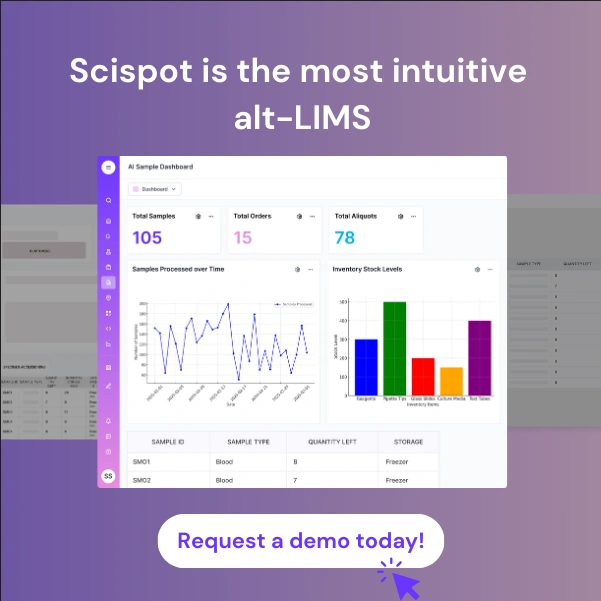
Case Example: Automating a GxP Workflow with AI
To concretely illustrate Scispot's capabilities, consider the case of a mid-sized biopharmaceutical company transitioning a lab process from R&D into a GxP-regulated workflow. This company needs to scale up production of a biologic therapy and must ensure GMP compliance in its quality control (QC) lab, while also seeking efficiency through AI-driven automation.
Scenario: The QC lab performs routine potency and purity assays on each batch of the biologic. Traditionally, analysts would run instruments (HPLCs, spectrophotometers, cell-based assays), then manually compile results, verify compliance with specifications, and prepare reports for batch release. This process was labor-intensive and prone to transcription errors. With Scispot, the lab automates and streamlines this entire workflow.
Real-time Instrument Data Capture: All QC instruments are integrated with Scispot's platform. As soon as a test is run, the raw data files (chromatograms, plate reader outputs, etc.) flow directly into Scispot, automatically associated with the correct batch record and test protocol. Analysts no longer manually enter results, the system captures readings from over a dozen instrument types in real-time. This not only saves time but ensures an unbroken data chain for compliance (each data point is timestamped and attributable).
AI-Assisted Analysis and Anomaly Detection: Scispot's AI layer then kicks in to help interpret and verify the data. For each assay, the AI Lab Assistant parses the results against acceptance criteria. If all is within spec, it can draft a summary report; if there are any out-of-spec or unusual patterns, it flags them immediately. For example, the AI might detect that a particular batch's potency trend is deviating from the last 10 batches and alert the team to a potential issue. In our case, during one run, the AI identifies a slight downward drift in potency readings that, while still in spec, might indicate an instrument calibration issue. The team is alerted proactively, allowing them to recalibrate the instrument before the next batch, potentially averting a future compliance deviation.
Streamlined GxP Compliance and Documentation: Because this is a GxP workflow, every action is logged. Scispot provides a full audit trail of the process, from data capture to AI analysis to any user decisions. Analysts and managers electronically sign off on the batch test results within Scispot, fulfilling 21 CFR Part 11 requirements for electronic records and signatures. The platform automatically compiles the batch report with all raw data, analysis, and approvals included, ready for regulatory inspection. What used to take days of paperwork is now largely generated by the system. If an auditor requests why a batch was flagged, the team can pull up the audit-trailed AI alert and resolution steps in seconds.
Results: The QC lab achieves a significant efficiency gain. Let's say previously analysts spent 4 hours per batch on data processing and reporting; with Scispot, this is cut by more than half, as much of the work is automated or accelerated by AI. Across dozens of batches, these savings free up entire headcount equivalents to focus on deeper analysis and process improvements instead of clerical tasks. In fact, labs using Scispot in production environments have reported reducing time spent on data management by up to 40%, while still improving compliance rigor.
Additionally, the early anomaly detection by AI leads to more robust processes, in our example, catching the drift early prevented a potential batch failure down the line. The company also benefits from having all batch data readily accessible for trend analysis, enabling continuous improvement in their manufacturing process.
This case exemplifies how Scispot blends flexibility, automation, and compliance in a real-world scenario. During development, the assays were developed in Scispot in a flexible R&D project space. As they moved to QC, the same platform enforced the SOPs and captured required compliance data. AI provided an assistive layer throughout, enhancing human decision-making rather than replacing it. Importantly, the lab's leadership maintained full oversight, dashboards in Scispot allow them to monitor KPIs like turnaround time and assay variability in real-time, which is invaluable for scaling up production.
Other examples abound: a molecular diagnostics lab might use Scispot to handle both exploratory biomarker research and CLIA-compliant diagnostic testing in one system, using AI to triage large genomic datasets. Or a biotech startup might manage their entire pipeline, from early experiments to IND-enabling studies, on Scispot, avoiding multiple point solutions and ensuring data continuity. In each case, Scispot's unified approach with AI and integration at its core enables labs to automate complex workflows that span scientific discovery and regulated operations, something legacy tools would struggle to achieve without extensive customization.
Conclusion: Future-Proofing the Lab with a Unified Platform
As laboratories navigate the rapidly evolving landscape of biotech R&D and production, the tools they rely on must evolve in tandem. The days of patchwork systems and one-size-fits-all legacy LIMS are numbered. Data-driven, AI-augmented, and compliance-sensitive labs require a new breed of platform, one that is as agile as early research demands, as reliable as regulated operations expect, and as open as modern data strategies require. Scispot exemplifies this new breed by combining what used to require multiple disparate systems into a single LabOS that can do it all: capture every data point, talk to every instrument, adapt to every workflow, and guide users with intelligent automation.
From an executive perspective, adopting Scispot is an investment in both innovation and efficiency. It empowers scientists and lab teams to work smarter with built-in AI and integrations, leading to faster discoveries and more streamlined processes. At the same time, it instills the rigor and control needed to confidently scale those discoveries into products with full compliance. The platform's modular, API-first architecture ensures that it can grow with the organization, avoiding the trap of technical debt or costly system swaps as the company expands. And with transparent pricing and data ownership, Scispot aligns its success with that of its customers, a refreshing break from the old vendor lock-in mentality.
In summary, Scispot is uniquely positioned as the ideal laboratory data platform for 2025 because it was architected around the very trends shaping the "lab of the future." Its API-first, data lake foundation is built for the era of big data and AI. Its comprehensive functionality (ELN + LIMS + SDMS + AI in one) is built for the convergence of research and development. Its openness and integrations are built for automation and avoiding silos. And its business approach is built for customer empowerment rather than dependency. For biotech CXOs and lab managers, this translates to greater agility in operations, more insight from lab data, and ultimately a faster path from lab bench to market, all while maintaining full control over one of their most valuable assets: their data.
As we move further into the decade, laboratories that leverage platforms like Scispot will be better equipped to handle whatever comes next, be it an order-of-magnitude jump in data volumes, a new AI breakthrough, or an unexpected regulatory challenge. By future-proofing their informatics infrastructure now, forward-looking labs can focus on scientific and business innovation, confident that their digital backbone will support their journey. Scispot, with its modern design and analytical prowess, offers that backbone, a laboratory platform not just built for today, but ready for the laboratory of tomorrow.
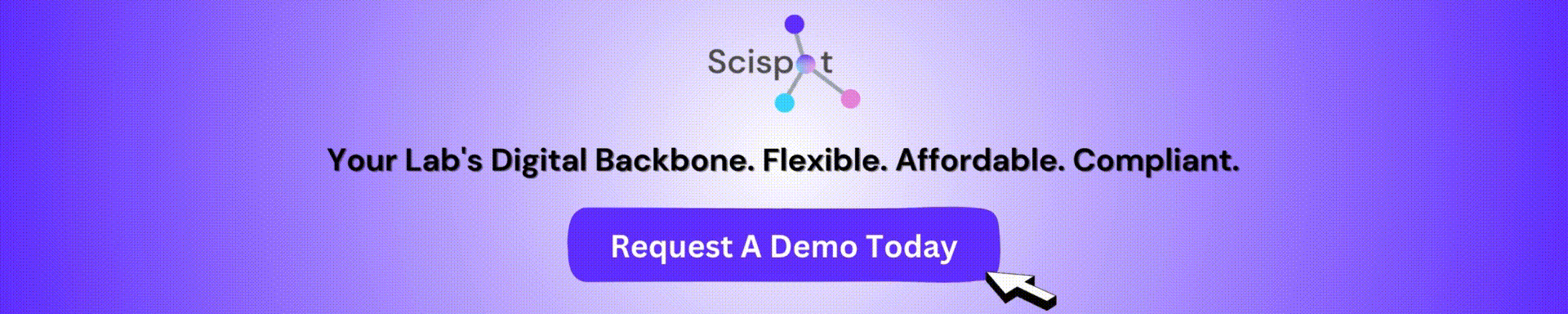