Biotech companies generate massive amounts of data—more than most other industries. This data is both a strength and a challenge, as much of it isn’t AI-ready and doesn’t actively make the company smarter.
In a recent interview on the Data in Biotech podcast by CorrDyn, Scispot founders Guru and Satya shared their vision of building a “digital brain” for every biotech. Imagine labs that learn with every experiment, every sample, and every result—bringing teams one step closer to breakthrough discoveries. This concept connects closely to the idea of a “digital twin in biotech,” where every experiment and result feeds into a digital model, creating a smarter, real-time version of the lab’s work.
This idea of a “digital brain” turns data overload into a unique advantage. Platforms like Scispot are leading the charge, creating a world where biotech companies learn from every step, build smarter workflows, and accelerate discovery through automation and seamless data integration. This vision of digital twin in biotech—and digital twin in pharma—could reshape how labs and companies drive discovery and innovation forward.
A Digital Brain for Biotech: What It Means and Why It Matters
At its core, a digital brain represents how biotech companies can seamlessly integrate data from wet labs, dry labs, external partners, and instruments—transforming it into actionable insights. Guru and Satya Singh, co-founders of Scispot, describe this as more than just storing experimental data; it’s a brain that learns and grows.
In the interview, Guru used a powerful analogy: just as a baby’s brain becomes smarter with each new experience, a digital brain in biotech evolves with every experiment, every sample, and every protocol. The more data it encounters, the “smarter” it gets, recognizing patterns much like a child learning language or relationships.
This evolution is especially crucial for early-stage biotech companies. Often, they begin with a few experiments and structured workflows. But as they expand, their needs change—they might start as contract research organizations (CROs) and later transition to in-house drug development. The ability to scale data infrastructure, connect teams effortlessly, and learn from every sample or protocol variation is what makes the digital brain concept so powerful. This aligns with the idea of a digital twin in biotech, where labs can mirror physical work in a dynamic, digital environment.
With a data lake at its core, Scispot allows companies to draw data from various sources—mass spectrometry, qPCR machines, even manual entries. This system’s flexibility means that companies can keep using existing tools like an ELN or LIMS, yet still leverage Scispot’s infrastructure to build a connected knowledge graph. This kind of biotech automation turns isolated data into a cohesive intelligence for the lab.
Imagine a scientist working on a Parkinson’s disease study. Every sample used, every result produced—it’s not just saved; it’s connected to a larger picture. The digital brain gets smarter, finding relationships between genes, proteins, and reagents, and even guiding the scientist’s next steps. This digital evolution, akin to a digital twin in pharma, is transforming how labs operate, turning data into insights that fuel discovery and innovation.
Why Real-Time Feedback Loops Matter
One of the game-changers of biotech data platforms like Scispot is the ability to generate real-time feedback loops. For example, if you’re running diagnostic tests in a molecular diagnostics company, each sample processed feeds back into the system, allowing smarter decisions based on cumulative knowledge rather than treating each test as an isolated event.
As Guru Singh mentioned in the podcast, biotech companies that embrace these feedback loops can evolve into data-first organizations. They can train their own AI models by leveraging years of accumulated experimental data to predict outcomes, suggest next steps, and even accelerate drug development timelines by years. This is where AI in biotech begins to unlock new potential, connecting past experiments to future insights.
For biotech companies, moving from traditional ELN/LIMS systems to a real-time feedback model can mean the difference between being just another testing company and becoming an industry innovator. As data becomes the most valuable asset, these companies aren’t just generating results—they are building a competitive moat around their proprietary data and AI models.
Externalization: The New Reality of Biotech
As biotech organizations increasingly externalize their research to CROs, academic partners, or cloud labs, the ability to integrate data from multiple sources becomes essential. One of the critical challenges that Scispot addresses is ensuring seamless inter-lab connectivity. Whether experiments are conducted in-house or by external partners, data flows into one unified platform where it can be analyzed, shared, and acted upon.
This capability is especially valuable for startups that lack the resources to build full-scale labs or tech stacks. By adopting a platform like Scispot, they can jumpstart their R&D without hefty infrastructure costs. They gain access to a customizable digital brain from day one, integrating effortlessly with partners and scaling with their needs.
Where Scispot Adds Value
While Scispot focuses on creating a unified digital brain, it goes even further by helping biotech organizations automate, templatize, and customize workflows from day one. The platform is crafted with a deep understanding of data flow within life sciences, whether it’s in research, diagnostics, or biomanufacturing.
Through AI-driven lab automation, Scispot enables companies to do more with less—a critical edge in an industry where every experiment, sample, and decision counts. Scispot’s biotech automation capabilities ensure that even small biotech startups can operate as efficiently as established players.
For instance, imagine running a complex molecular diagnostics workflow. With Scispot, all steps—from sample prep to results—can be automated, tracked, and seamlessly linked to inventory management, ensuring that every reagent is accounted for. This automation extends to cost tracking, compliance monitoring, and even sample shipment, significantly reducing errors and manual workload.
But Scispot truly shines in its scalability. As companies grow, workflows evolve, becoming more complex and interconnected. Scispot adapts to these changes, offering customizable data models, real-time alerts for inventory or equipment issues, and integrations with existing systems like ELNs, LIMS, and other tools. This adaptability is essential for biotech companies aiming to scale quickly without compromising data quality or integrity.
The Future: Data as the Primary Asset
Both AI in biotech and biotech automation are founded on the belief that data is biotech’s most valuable asset. The future lies in companies becoming data-driven, leveraging AI to gain insights faster, and ensuring that every experiment not only fulfills its immediate purpose but also contributes to a growing digital brain.
Platforms like Scispot are leading this transformation by enabling companies to integrate, automate, and optimize their processes. In an industry where speed, accuracy, and innovation are everything, tools like these help biotech companies not only survive but thrive.
So, whether you’re an early-stage startup or a more mature organization, it’s time to rethink your approach to data. Start building your digital brain today.
Final Thoughts
In today’s rapidly evolving biotech landscape, companies that don’t embrace AI in biotech and biotech automation risk falling behind. Whether you’re just starting or scaling up, platforms like Scispot offer the tools to build smarter processes, automate workflows, and ultimately accelerate innovation. The question is no longer if you should adopt these systems—but how soon you can get started.
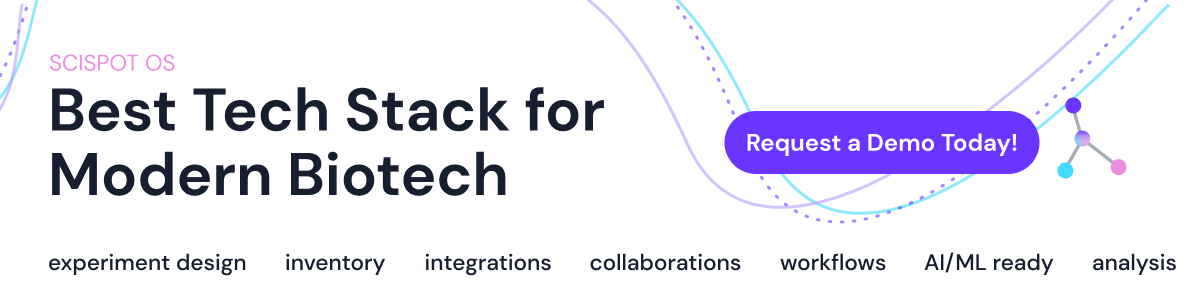