In the rapidly advancing field of proteomics, the need for effective and user-friendly data management tools is becoming increasingly imperative. Enter Scispot, a pioneering platform designed to track, automate, and visualize the full metadata journey in proteomics workflows. Our offering spans the comprehensive journey of proteomics data from working compounds and cell lines, all the way to individual experiment runs.
Defining The Importance of Proteomics
Proteomics is the study of proteins, their structures, functions, and interactions within a biological system. It involves the comprehensive analysis of all the proteins expressed by an organism or a specific cell type. Proteins are vital molecules that carry out a wide range of biological functions, such as enzymatic activity, signaling, and structural support. Understanding proteomics provides insights into the mechanisms underlying diseases, drug discovery, and personalized medicine. It enables the identification of disease biomarkers, the characterization of protein modifications, and the exploration of protein-protein interactions, contributing to advancements in various fields, including biology, medicine, and biotechnology.
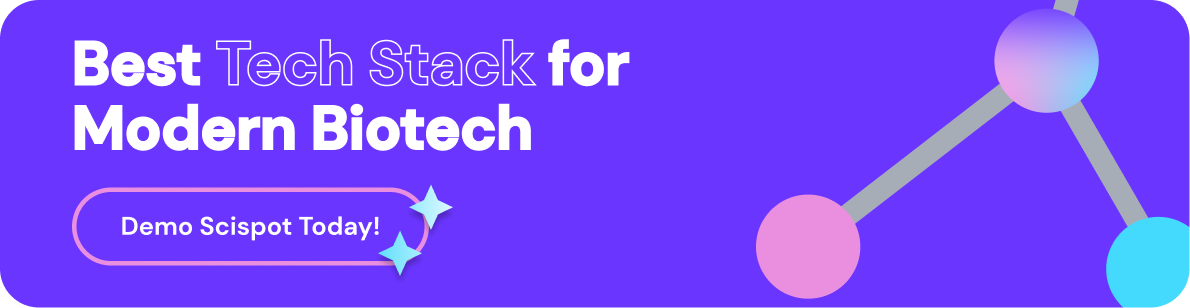
Proteomics Analysis Tools and Features for Streamlined R&D
Scispot is a comprehensive platform for proteomic analysis, offering advanced algorithms and workflows for protein identification, quantification, and statistical analysis. It enables researchers to uncover differentially expressed proteins and perform label-free or isotopic labeling-based quantification. Scispot facilitates targeted proteomics analysis by assisting in experiment design, optimization, and selective quantification of specific proteins and peptides of interest. Integrated data analysis features include statistical tools, clustering algorithms, pathway analysis, and data visualization, aiding in the identification of significant protein expression changes and exploration of biological pathways. It covers protein identification, quantification, PTM analysis, and protein network analysis, integrating with mass spectrometry platforms and search algorithms. The platform incorporates lab informatics resources for protein-protein interaction analysis and functional network exploration. Furthermore, Scispot specializes in efficient data management throughout the proteomics workflow, featuring intuitive interfaces for sample recording, scanning, and metadata capture, ensuring accurate records and enhancing reproducibility.
Experiences Seamless Data Integration and Visualization in Your Computational Lab with Scispot
Proteomics analysis tools are crucial for computational labs for several reasons. Firstly, these tools enable computational scientists to process and analyze large-scale proteomics datasets efficiently, which would be impractical to handle manually. Secondly, they provide advanced algorithms and statistical methods for protein identification, quantification, and analysis, facilitating the exploration of complex biological systems. Thirdly, proteomics analysis tools help in the interpretation of experimental results, aiding in the identification of differentially expressed proteins and the discovery of meaningful patterns and relationships. Fourthly, these tools allow computational scientists to integrate proteomics data with other omics datasets, enabling multi-omics analyses and systems biology approaches. Lastly, proteomics analysis tools support the development and validation of computational models and algorithms, enhancing the predictive capabilities of computational approaches in proteomics research.
For computational scientists, Scispot opens a world of seamless programmatic interactions. Leveraging our platform, they can not only create and manipulate plates and samples programmatically but also tap into the robust metadata API for downstream analysis. The integrated knowledge graph of metadata, connecting from cell lines to runs, allows for improved data connectivity, accessibility, and exploration, fostering a rich environment for hypothesis generation and testing.
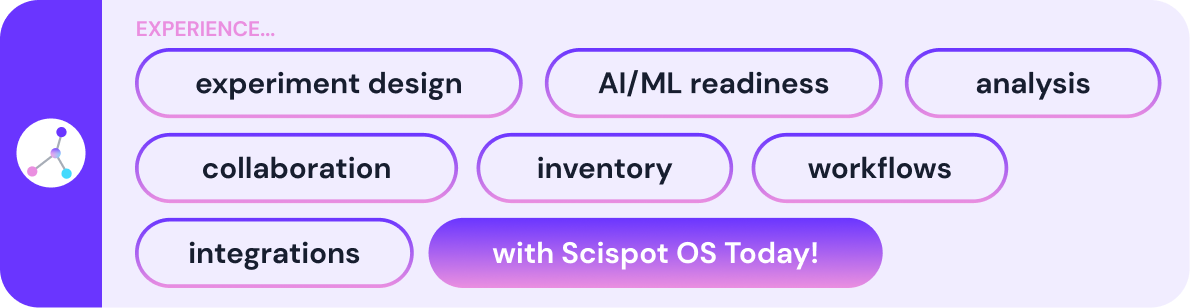
Scispot Enhances Sample Management and Research Efficiency in Proteomics Wet Labs
Scispot offers an intuitive interface specifically designed to meet the needs of wet lab scientists in proteomics research. The platform simplifies the recording and scanning of samples, plates, and associated metadata, ensuring easy and accurate data capture for sample submissions. By providing an efficient and user-friendly interface, Scispot reduces the burden of data management overheads, allowing scientists to focus more on their experiments. The streamlined data recording process enhances the accuracy and completeness of records, contributing to the reproducibility of experimental outcomes. Overall, Scispot empowers proteomics labs by optimizing data management practices, facilitating smooth workflows, and promoting reliable research results.
Here is an example of the knowledge graph that Scispot helps you build for your proteomics metadata:
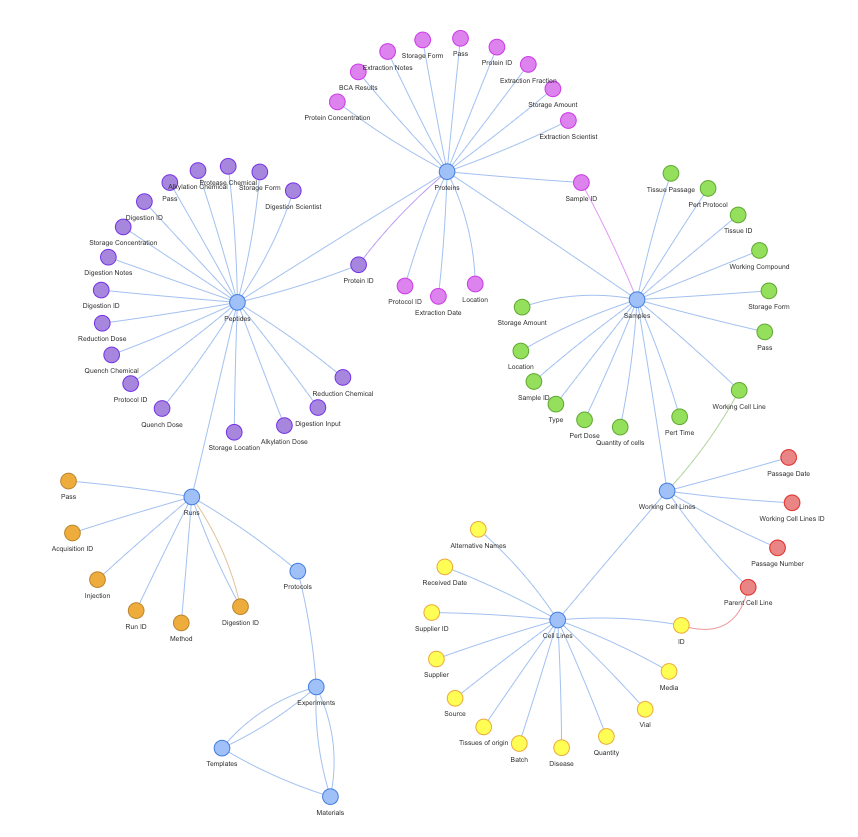
Scispot for Proteomics Companies
Scispot is the ultimate choice for your proteomics research needs. Built by scientists for scientists, our platform combines advanced algorithms and workflows to provide powerful tools for protein identification, quantification, and statistical analysis. Trusted by hundreds of biotech researchers, Scispot offers a comprehensive suite that covers all aspects of proteomic data analysis, from PTM analysis to protein network exploration. With user-friendly interfaces and intuitive features, Scispot streamlines data management, ensuring accurate and complete records, ultimately accelerating your research and enabling groundbreaking discoveries. Request a demo today.
Here's what Users have to Say about Scispot's Proteomics Tools
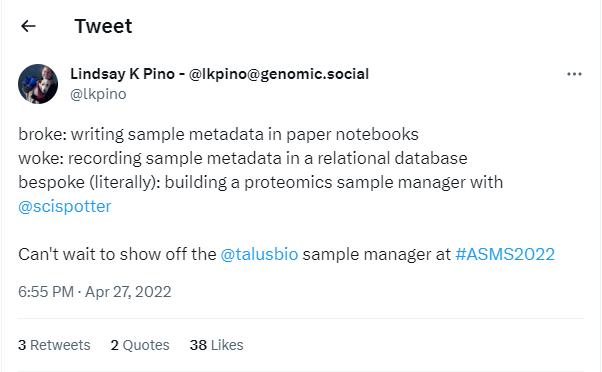