The Future of Hybrid Biotech: Key Takeaways from Our Live Event
The biotech industry is advancing rapidly. A decade ago, most companies relied on a wet lab vs dry lab approach, keeping computational and experimental workflows separate. Today, hybrid biotech lab integration is changing the game by combining computational predictions with real-world biological validation. Companies like Recursion Pharmaceuticals and Schrodinger use computational tools to screen millions of molecules before conducting wet lab testing, dramatically reducing time and costs in drug discovery and biomarker research.
On December 19, 2024, Scispot hosted a LinkedIn Live event titled Dry-Lab-First vs. Wet-Lab-First: The Hybrid Biotech Evolution and Its Future. Our co-founder, Satya, led an engaging discussion on AI-driven wet lab validation, data infrastructure in biotech, and integrating wet and dry labs. The event attracted biotech professionals, researchers, and data scientists eager to understand the evolving landscape of computational biology and experimental validation.
Key Insights from the Event
The Shift from Wet Lab-First to Hybrid Biotech Models
Historically, biotech companies followed a wet lab-first model, relying heavily on experimental data. Now, computational biology is leading innovation, making hybrid biotech models the new standard.
- Dry labs optimize experiments with computational tools, reducing costs and improving efficiency. Companies like Insilico Medicine use predictive models to identify promising drug candidates before wet lab validation, minimizing expensive trial-and-error processes.
- Wet labs validate computational predictions, ensuring accuracy before real-world application. Moderna, for example, used computational modeling to optimize its mRNA vaccine before lab synthesis, significantly accelerating development.
- A hybrid approach enhances hypothesis validation and generates reliable data-driven insights. The Human Cell Atlas project integrates computational models with experimental biology to refine our understanding of human cell types.
- Researchers are embracing multi-omics data integration, combining genomics, transcriptomics, and proteomics to refine computational models and ensure accuracy in wet lab validation. Scispot’s genomics solution supports this integration.
The Role of Computational Tools in Biotech Innovation
Computational methods are revolutionizing biotech, offering faster insights and smarter research strategies.
- Tools like Schrodinger’s platform predict molecular interactions before wet lab testing, allowing scientists to focus on high-probability candidates.
- Computational modeling reduces failed experiments and speeds up research. DeepMind’s AlphaFold has transformed protein structure prediction, making drug discovery more efficient.
- Improved predictive models and lower computational costs are making hybrid biotech models more accessible for startups and established labs alike.
- Machine learning algorithms help researchers analyze datasets quickly, cutting down the time needed to identify viable drug candidates.
- AI-powered high-throughput screening enables researchers to filter ineffective compounds before they reach the lab bench, optimizing efficiency.
Building the Right Data Infrastructure
Handling and integrating large datasets is one of biotech’s biggest challenges. Data infrastructure in biotech ensures smooth collaboration between computational and experimental teams.
- Hybrid labs require structured data management to maintain accuracy and consistency. The NIH’s BioData Catalyst platform supports large-scale computational research, allowing for better data analysis.
- Automation plays a key role in modern biotech workflows. AI-driven tools streamline experiments and improve data integration.
- Scispot’s API-first platform bridges the gap between computational and wet lab workflows, ensuring smooth data transfer and reducing silos.
- Data standardization is crucial for improving collaboration between computational and experimental teams, preventing discrepancies in research outcomes.
- Cloud-based data repositories are becoming essential, enabling secure data access and analysis across global biotech teams.
Real-World Applications of Hybrid Labs
Satya shared several examples of companies successfully using hybrid biotech models to boost efficiency and innovation.
- Computational-first biotech startups are implementing wet lab vs dry lab workflows to validate experiments. Absci, for instance, uses AI-powered tools to design proteins before synthesis, cutting costs and increasing efficiency.
- Wet lab-first companies are integrating computational tools to improve modeling and analysis. Eli Lilly refines drug formulations using simulations before conducting wet lab experiments, leading to faster development timelines.
- Companies like Amgen and Schrodinger are adopting hybrid models, balancing computational tools with wet lab validation to enhance accuracy and efficiency.
- Researchers are utilizing synthetic biology to generate highly precise datasets for computational modeling, ensuring better integration between AI-driven predictions and experimental outcomes.
- Biotech firms are leveraging robotic lab automation to streamline repetitive wet lab tasks, reducing human error and increasing precision.
The Future of Integrating Wet and Dry Labs
The biotech industry is shifting toward automation and seamless integrating wet and dry labs workflows. This transformation is driven by increasing access to computational tools, AI-powered predictions, and automation technologies.
- Robotics and machine learning are enhancing wet lab precision and efficiency. LabGenius, for instance, uses robotic platforms to automate complex lab procedures, optimizing protein engineering.
- Data-driven feedback loops are accelerating research. Companies like Recursion use AI-powered automation to speed up disease model validation, providing quicker insights into potential treatments.
- Computational biologists and wet lab scientists must collaborate using shared workflows to ensure consistency in data interpretation, improving research accuracy.
- Future biotech research will likely integrate personalized medicine approaches, using AI to customize treatments based on individual genetic profiles, improving patient outcomes.
- With AI and automation advancing rapidly, biotech firms are exploring self-learning lab systems that adapt and refine experiments in real time, boosting accuracy and efficiency.
Conclusion: The Rise of Hybrid Biotech Labs
Biotech is moving toward a future where wet labs and dry labs work together seamlessly. Stronger data infrastructure in biotech, advanced computational tools, and increased automation are driving this change. Companies that embrace this approach will be better positioned to accelerate research, lower costs, and achieve groundbreaking scientific discoveries.
If you missed the event, stay tuned for more discussions on hybrid biotech lab integration, computational modeling, and the latest tools shaping biotech’s future.
Interested in how Scispot can help your biotech company adopt a hybrid biotech model? Get in touch with us today!
Watch the Webinar Recording
Missed the event? You can watch the full recording on YouTube:
Let’s build future of hybrid biotech together!
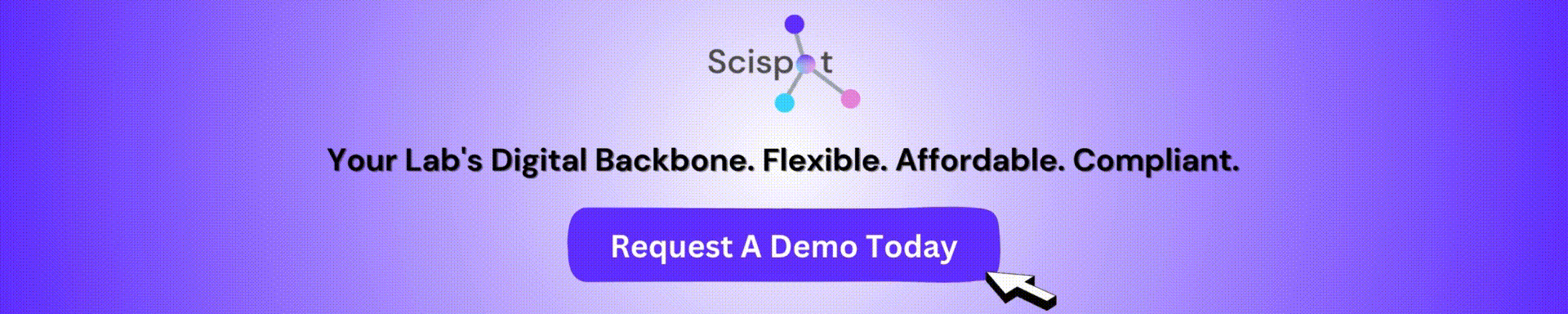