2024: From data infra to AI agents
AI agents have become indispensable in industries like logistics, finance, and healthcare. Yet, biotech labs—the backbone of scientific progress—have lagged, held back by fragmented systems and manual processes. A hidden tab in a spreadsheet wiped $12 billion off Amgen’s market cap, starkly illustrating the cost of relying on outdated methods.
2024 was the year where biotech and lifescience industry acknowledged the importance of AI agents. Scispot's 2024 roadmap heavily invested in making such AI agents accurate aligning with outcomes lab leaders' look for while investing in a 'system of record' tools. Scispot is the middleware platform for lifescience labs that acts as a 'system of record' for sample centric and high throughput labs. As a result, it reduces friction between wet lab and dry labs. A key area of focus is structured & unstructured data and integration - helping Biotech AI companies create the data infrastructure and workflows to enable AI and analytics in R&D and manufacturing.
The core elements of Scispot OS include several transformative tools:
- Labsheets: For those familiar with Benchling’s registries, think of Labsheets as an API-first graph database tailored for wet lab scientists. Labsheets enable users to build comprehensive lineages of samples, inventories, instruments, and experiments. Essentially, it serves as a structured data lake, harmonizing data from disparate sources into a single, unified repository.
- Labspaces: More than just a digital lab notebook, Labspaces integrates hundreds of third-party data types, including images, chemicals, proteins, and databases. It’s designed to streamline the collation and contextualization of experimental data, making it an indispensable tool for lab documentation.
- GLUE: The lynchpin of Scispot OS, GLUE connects all these elements into a cohesive ecosystem. Built on an API-first platform with robust instrument connectors, it ensures seamless integration between data, workflows, and lab operations.
.png)
In 2024, Scispot introduced a new dimension to this robust infrastructure: AI agents. With an already-established data framework designed for high-throughput, sample-centric labs, Scispot focused on leveraging AI agents to significantly enhance lab productivity. By addressing operational inefficiencies and automating repetitive tasks, these agents allowed scientists to concentrate on research and innovation.
Scispot introduced the world’s first alt-LIMS AI agent, designed to fundamentally change how biotech labs function. Unlike generic AI retrofitted for scientific workflows, these agents tackled the specific pain points of modern labs—fractured data systems, inefficient processes, and relentless compliance hurdles. With a unified system of record at their core, Scispot’s AI agents harmonized data streams from instruments and workflows, dissolving silos and enabling streamlined operations.
The impact was transformative. Labs experienced a surge in productivity as these agents automated labor-intensive tasks, significantly reduced manual errors, and provided real-time insights essential for critical decision-making. Beyond just operational efficiency, Scispot’s AI agents accelerated discovery timelines and ensured precision compliance with industry standards. For scientists, it wasn’t just about faster workflows; it was about having tools that evolved alongside the complexity of their research.
Why AI Agents Matter in Labs
In labs, operational inefficiencies often overshadow scientific innovation. Hours are spent setting up plates, managing isolated data, and navigating systems incapable of interoperability. Scispot’s AI agents were developed to address these bottlenecks, enabling researchers to redirect their focus toward meaningful discovery.
Consider Plato, one of Scispot’s AI agents, which has redefined how biotech labs handle high-throughput workflows. Before Plato, researchers spent countless hours manually designing multi-well plates, assigning controls, and entering metadata into disconnected systems. Each step presented opportunities for errors that could derail entire experiments and waste valuable resources. Even after data collection, scientists faced another bottleneck: cleaning and organizing raw outputs for analysis, often requiring days of additional effort.
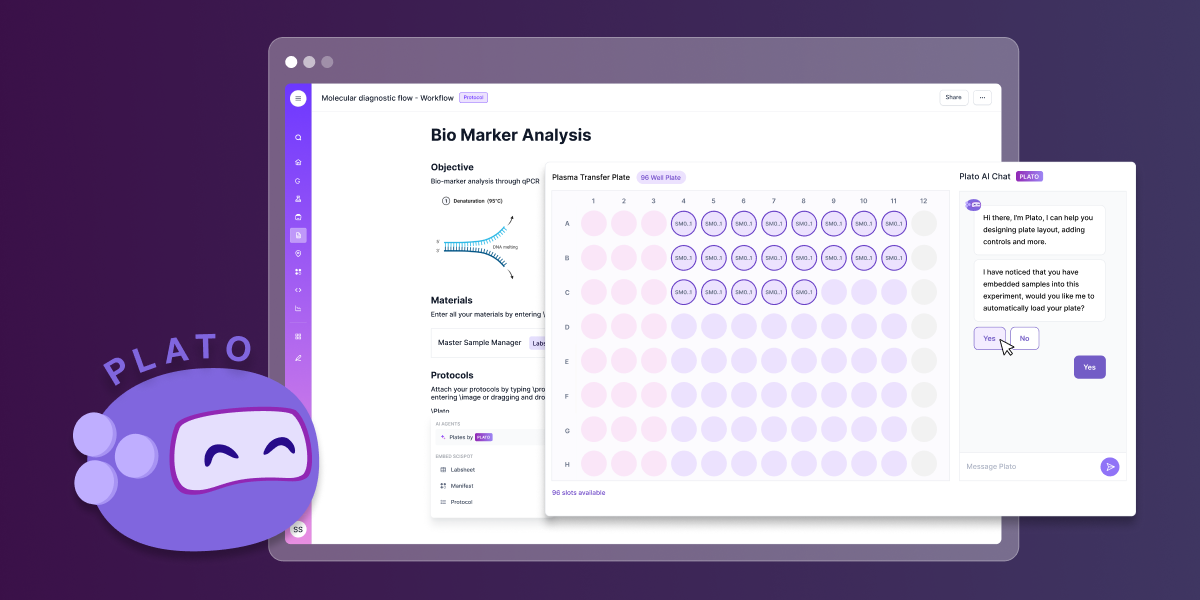
With Plato, these inefficiencies became a thing of the past. Researchers can now simply input experimental parameters, and Plato automates the plate design, ensuring controls and standards are properly assigned. Metadata is synchronized seamlessly with connected instruments, preemptively flagging potential issues before experiments even begin. Once the data is collected, Plato processes and organizes it automatically, delivering clean, ready-to-analyze datasets in real time.
This transformation goes beyond operational improvements; it fundamentally changes how labs approach experimentation, enabling faster insights and reducing costly errors at every stage of the process.
Lessons from Other Industries
Lifescience labs may have been slower to adopt AI, but its transformative impact is undeniable when looking at other industries:
- Logistics: Amazon employs AI to forecast demand, optimize delivery routes, and streamline warehouse operations, enabling rapid scalability and precision.
- Finance: Firms like BlackRock leverage AI to process and analyze billions of data points, empowering real-time, high-stakes decision-making.
- E-Commerce: Companies like Shopify deploy AI agents to enhance online shopping experiences. These agents provide personalized product recommendations, manage inventory levels, and even handle customer queries in real-time. For example, AI agents analyze browsing behavior and purchase history to suggest relevant products, increasing conversion rates while simultaneously automating customer support for a seamless shopping journey.
These industries showcase a vital truth: AI is no longer optional for scaling—it’s essential. Scispot drew inspiration from these successes, tailoring automation for biotech labs to solve challenges unique to the field. The question isn’t whether automation can work in biotech, but how many labs will left behind if they don't embrace it.
From Chaos to Clarity: A Case Study
A diagnostics lab in South San Francisco specializing in liquid biopsies exemplifies the impact of Scispot’s approach. Processing thousands of samples monthly, they faced significant challenges: a rigid LIMS system that couldn’t scale, instruments operating in silos, and compliance reporting that took weeks.
After implementing Scispot, the transformation was remarkable:
- Plato automated multi-well plate workflows, saving over 2,000 hours annually.
- Scispot’s GLUE API enabled seamless integration of instruments like Hamilton liquid handlers and LCMS systems, reducing errors by 40%.
- Compliance reports, once a multi-week endeavor, were completed in minutes.
The result? Patient report turnaround times dropped from five days to two, accelerating critical diagnostics and saving lives.
Scispot’s 2024 Roadmap
Each quarter of 2024 brought innovations on top of Scispot OS. The focus in 2024 was to leverage Scispot's data infrastructure to seamlessly build AI agents for productivity of Scispot's customers.
Quarter 1: Automation Foundations and Seamless Compliance: Scispot didn’t just hit the ground running—it rebuilt the track. Q1 saw the introduction of GxP controls for unstructured data, a lifesaver for labs wading through regulatory minefields. Add to that detailed chain-of-custody tracking to keep every sample’s journey crystal clear, and you’ve got compliance handled before your morning coffee.
The GLUE computational & API features expanded to include plate readers, LCMS, and other critical instruments, reinforcing Scispot as the ultimate lab middleware. And, for good measure, Scispot added 6,000+ Zapier apps to its integration arsenal. Whether you’re syncing lab data with Slack, Google Sheets, or your team’s productivity tool of choice, Scispot became the undisputed champion of connectivity.
Quarter 2: Making Data Speak Human: Lab dashboards got a brain upgrade in Q2. Scispot unleashed NLP-powered dashboards, making lab data visualization as intuitive as asking, “What’s the throughput on Protocol B last week?”—and getting answers in seconds.
This was also the quarter where Scispot’s AI Agents were born. Think of features like PDF-to-protocol conversion (no more retyping) and embedding search architecture (find buried data faster than ever). Scispot didn’t stop there: it rolled out bulk updates for Labsheets, slashing manual work by a whopping 60% across workflows.
Quarter 3: The Age of AI and Precision Connectivity: The biotech world got a new favorite lab assistant: Plato, Scispot’s AI for multi-well plate design and analysis. Plato redefined precision in high-throughput screening workflows, ensuring not a single well was wasted. For computational scientists, Q3 delivered JupyterHub integration, enabling real-time script execution within workflows. No more context-switching or late-night troubleshooting. Plus, Scispot took barcode scanner integration up a notch, ensuring smooth sample traceability every step of the way.
Quarter 4: AI-Driven Reporting and Diagnostics Powerhouse: By Q4, Scispot’s AI Agents leveled up. They didn’t just harmonize instrument data—they learned from your workflows to deliver customer-specific insights. Whether it was automating QC for mass spec runs or handling messy instrument data, these agents became indispensable.
Structural biology labs rejoiced with the introduction of MRC and PDB data visualizations, making it easy to embed complex insights directly into Scispot. And for diagnostics teams, multi-well plate integrations with liquid handlers and plate readers brought automation to a new level, speeding up assays and ensuring every step was traceable.
.png)
Why Labs Need AI Agents Now More Than Ever
Let’s face it: labs have been playing catch-up with other industries for years. While e-commerce, finance, and even healthcare admin have automated their grunt work, labs are still stuck in the digital equivalent of the Stone Age. Think sprawling spreadsheets, disconnected instruments, and workflows that scream “Do it again because someone missed a step.”
But here’s the thing—science can’t afford to stay stuck. Labs are the engine rooms of biotech innovation, and inefficiency isn’t just frustrating; it’s holding back breakthroughs. That’s where AI agents step in, not as a luxury, but as the lifeline labs have been waiting for.
What's next?
2025 is set to be a big year for lab automation. The way labs connect their instruments and software is about to change in ways we’ve only imagined. No more clunky systems or manual workarounds—labs are demanding seamless, smart connections that don’t just get the job done but actually make it easier.
The whole idea of middleware is being redefined. We need more API-first platform to leverage the benefits of AI.
This is exactly where Scispot comes in. We’re not just following the trend; we’re shaping it. From integrating instruments like liquid handlers and mass specs to equipping labs with AI agents that handle the tedious stuff, Scispot is inventing the standard for what a modern lab should look like.